Why Data Quality Is the Load-Bearing Wall of Your Data Analytics Architecture
Why Data Quality Is the Load-Bearing Wall of Your Data Analytics Architecture
If you’ve ever undertaken a home remodeling project, you’re likely familiar with the importance of load-bearing walls. A load-bearing wall is a structural element that supports the weight of the elements above it. Remove the load-bearing wall, and the structure crumbles.
Data analytics also involves a load-bearing wall, and that’s data quality. Without high-quality data, analytics, reporting, and forecasting- all become suspect.
For government organizations, the negative consequences can include missed budgets, erroneous interpretation of trends, inaccurate predictions, and inadequate constituent services. Above all, poor data quality inhibits state and local government’s ability to deliver positive outcomes for the people they serve.
What you need, then, is an effective way to ensure the quality of your data. But to realize that goal, you need to understand the factors that contribute to high-quality data and commit to a formal data governance framework.
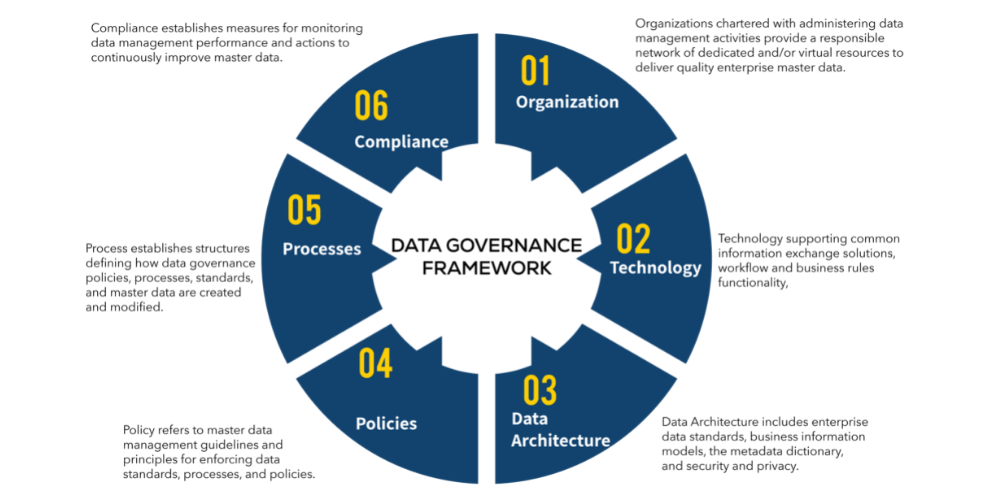
Data Quality for Tangible Value
As data feeds proliferate, data quality can erode at various points – up to the moment of output. If data is “dirty” – that is, if it’s inaccurate, incomplete or inconsistent – then any value derived from the data is constrained, and decision-making is diminished.
Poor data quality can have a direct impact on an organization’s bottom line. A recent Gartner report found that poor data quality costs organizations about $12.8 million a year.
Just what constitutes “clean,” or high-quality data? As a starting point, the data must be accurate, legitimate, timely, fit for purpose and in a usable format. In addition, consumers of the information should perceive the data to be trustworthy, applicable, clear and available when it’s needed.
Maintaining Data Quality with a Data Governance Framework
A formal data governance framework is the most efficient, reliable and effective way to achieve data quality. Such a framework brings together business and IT stakeholders to define organization-wide rules to maintain data integrity, security and quality.
Your data governance framework should touch every aspect of data management, from the underlying technology to policies around how you generate, capture, secure, retain and use data. It should also include mechanisms for ensuring regulatory compliance and metrics for measuring whether your framework is delivering the outcomes you expect.
Start by performing an inventory of your data, including where it’s located – for example, on-premises or in the cloud – who “owns” it, and what standards and processes are in place for securing and handling it.
Then, address key data governance drivers:
- A common data standard – Create a set of standard rules around each data element to ensure data remains consistent and of high quality.
- Data security and privacy – You can’t achieve enterprise-level cybersecurity – or hope to meet compliance obligations – without governance.
- Data interoperability – If you expect to conduct useful data analytics, you need a seamless and controlled exchange of information among relevant applications. Governance is essential to maintain data structure and ownership as data flows across connected systems.
- Data access and consumption – Create a data governance model that reinforces the integrity of the data and ensures that all information is easily (yet securely) sharable and consumable.
Data Quality Actions to Take Now
Implementing a data governance framework is essential to maintaining data quality, but it’s not the only way to achieve data analytics maturity. You can also take these actions to position your organization to begin benefitting from quality data:
- Invest in expertise – Establish a team dedicated to the collection and management of data throughout your organization.
- Audit and cleanse – Routinely review and rationalize your datasets to make sure your data remains timely and relevant.
- Model and maintain – Practice continuous data modeling so that your data feeds and data stores adapt as your organization’s needs change.
- Become a data quality leader – Implement an analytics center of excellent to turn your organization in an operation truly driven by high-quality data.
Data is a strategic asset for the public sector. Having high-quality data and analytics can help agencies uncover new ways to optimize operations, deliver services and a drive positive outcomes for constituents.
Voyatek Team