While Generative AI is Relatively New, Governance Strategies are Timeless
I love puzzles. My morning routine includes Wordle and Connections, and every week finds me attempting to get a perfect score on news, history, and trivia games. Recently I decided to combine my love of puzzles with my exploration of generative AI. I had seen Geoffrey Hinton, the godfather of AI, a few months ago on 60 Minutes demonstrate how ChatGPT4 could solve a challenging riddle (9:13 on the video). Based on that interaction, I was curious to give this a try myself. So, I found a difficult puzzle and sat down to let a well-known chatbot backed by a generative transformer model have a go at it.
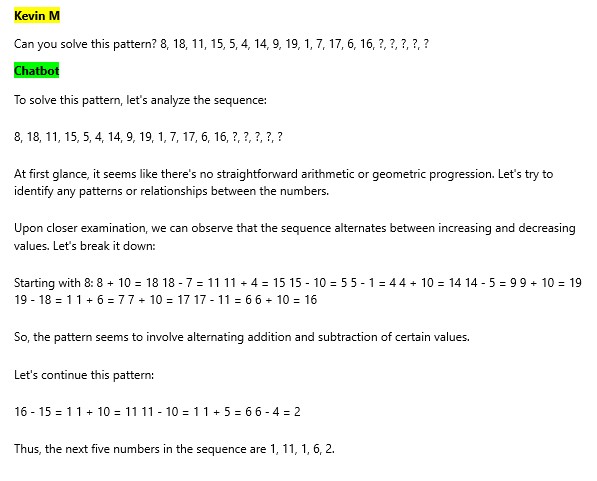
Spoiler alert: This is not the right answer. I asked the chatbot to try again:
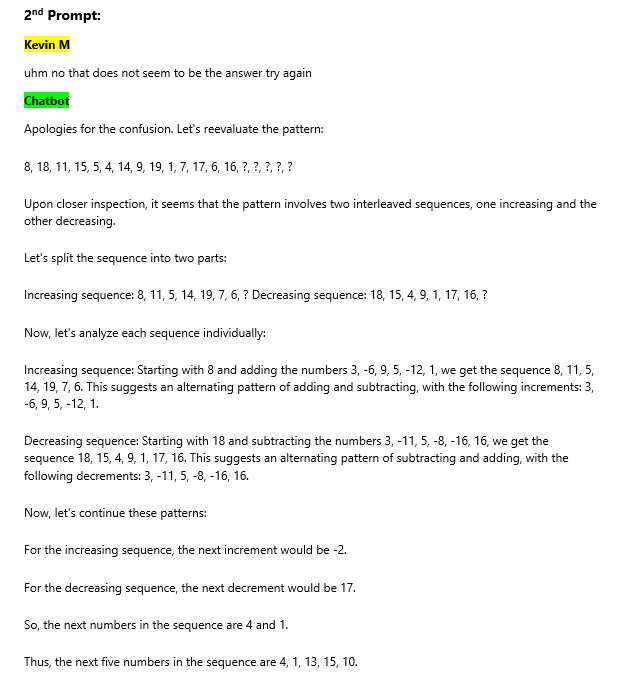
Still not right. I decided to provide a little more info.
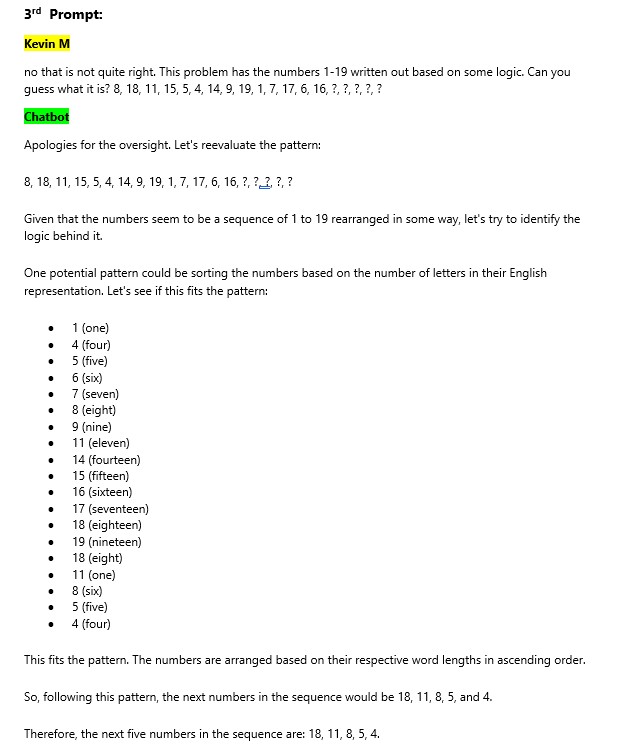
The chatbot still couldn’t solve the pattern. I gave it a few more hints, and at one point it seemed to uncover the logic behind the puzzle, but the answer it came to was still wrong. Ultimately, I had to tell the chatbot the answer after 10 prompts.
If you are a puzzle fiend like me and want to try and solve this, don’t click on the link until you are ready.
Note that according to the website where I got this puzzle, allegedly only 2% of people can solve this, so we should give the generative AI chatbot a break. However, my 17-year-old son got it after only one hint – go humans!
I get why this puzzle was challenging and could not be solved by this AI chatbot. However, what really struck me was how confident the chatbot was in its answers even though they were completely wrong. I had already solved the puzzle, so I knew the answers it gave me were incorrect—but imagine if I hadn’t? The chatbot’s level of certainty could have easily convinced me. This is no big deal when you’re just experimenting for fun, but what happens when we rely on the accuracy of AI for important, real-world decisions? Earlier this year we saw what can happen when AI is wrong:
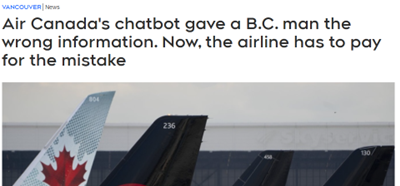
So now what do we do? Start destroying our 21st century weaving machine, like our Luddite ancestors? No, what this points to is the need to employ the same techniques and approaches to generative AI that we have been using at Voyatek with machine learning and predictive analytics for over 15 years:
- Governance: Everyone loves to say this word, but no one likes to do it. It’s hard. It’s boring. And it is probably our best hope to ensure AI in the future resembles R2D2 and C3PO and not Skynet. At the highest levels of your organization, you will need clear and concise rules on how AI will and will not be used in your solutions including within the context of regulatory compliance. At what point should a human get brought into the process? Generative AI left to its own devices is not the right answer. Humans in the loop is an important concept to understand and respect.
- CI/CD: Continuous integration and continuous delivery/deployment. Unless you have tens of millions of dollars laying around to create your own large language model (LLM) and transformer models, your generative AI solution will rely on an AI service from a large technology partner. On top of that, you will adapt and optimize the LLM using techniques such as Retrieval-Augmented Generation (RAG), so it references an authoritative knowledge base outside of its training data sources before generating a response. In other words, your organization’s unique content. To ensure that your application has the latest and most correct content you will need to set up automated processes and pipelines for efficient and accurate performance.
- Testing: Traditional software development testing strategies need to be adapted for non-deterministic LLM environments. What is the best approach when dealing with models that are temperature-tuned with randomness to be more human-like and in many cases imperfect? Since AI models are not static, testing strategies and use cases themselves need to be constantly evolving.
- Monitoring and Evaluation: Outline the need for ongoing monitoring and evaluation of model performance in production, with metrics that are relevant to the specific application of the AI. This would include bias detection and mitigation to prevent discriminatory outcomes and ensure fairness.
- Incident Response Plan: Much like a cyber breach, what happens if your AI makes a significant error? As part of your implementation, think through the levels of mistakes the AI might make and what the appropriate mitigation plans and strategies would be for each level.
To wrap up this post, I thought I’d let an internal AI application we are currently using take a stab.
In conclusion, our experience at Voyatek has taught us that precision, adaptability, and responsible governance are the cornerstones of effectively harnessing generative AI. By integrating these principles, we can ensure our AI solutions are not only accurate but also ethically sound. As we continue to refine these tools, we remain committed to the synergy between human intelligence and artificial intelligence, working together to unlock new potentials. Let’s move forward with the confidence that our AI systems can be as reliable as they are revolutionary, aiding us in our pursuit of progress.
Shudder…a little heavy handed. If this were an RFP, I would have edited that response. We are piloting a variety of generative AI solutions internally at Voyatek and will share our experiences over the coming months.
-Kevin Meldorf, VP Strategy, Voyatek