Predictive Analytics in Healthcare: Three Lessons Learned
Predictive Analytics in Healthcare: Three Lessons Learned
Predictive analytics has come a long way over the past decade. Ten years ago, predictive analytics were rudimentary, at best; today, they’re instrumental in improving healthcare and societal outcomes. For proof, look no further than how predictive analytics have been used to navigate the COVID-19 pandemic.
Although predictive analytics is all about the future, we can gather important insights from past analytics efforts. In particular, an ambitious Camden, N.J. program initiated more than 10 years ago can teach us some valuable lessons about how healthcare organizations should–and should not–use predictive analytics.
Camden’s “hotspotting” program attempted to use predictive analytics to determine which patients were driving up healthcare costs unnecessarily. The idea was to use past patient data to make better determinations about a patient’s likelihood for hospitalization and provide more targeted (and, therefore, more cost-effective) care.
Recently, however, the effectiveness of the “Camden Coalition” approach has come into question. Research has shown that the program has had a negligible impact on decreasing hospital readmissions, undercutting the foundational goal of the effort. Turns out, when the coalition was created, the only data being taken into consideration was patient data. It failed to take into account data regarding crime, homelessness, and other significant factors that contribute to the overall health of the community.
The Camden exercise has, therefore, taught us some valuable lessons when it comes to predictive analytics in healthcare:
The term “predictive analytics” is somewhat of a misnomer
The word “predictive” implies the ability to see the future, which of course we cannot do. Even with a vast amount of patient data at our disposal, we cannot say with 100 percent certainty that an individual will succumb to cancer, for example.
We can, however, use historical data to make a reasonably accurate assessment that allows us to determine the likelihood that something will happen. For instance, we can take a look at the physician population within a particular county and see which of those physicians have traditionally over prescribed painkillers. Based on that information, we can state with a fair amount of certainty that those physicians have and will continue to contribute to a state’s opioid epidemic. We can then work with law enforcement to target those physicians as a group effort to stem the tide of the state’s opioid crisis.
The Commonwealth of Virginia’s FAACT program is a successful example of this approach. There, the state is pooling data and resources from different agencies to provide a complete picture of opioid use and its contributing factors. It shows how predictive analytics, done correctly, can have a positive impact on people’s health.
Data cannot be siloed
FAACT also shows the value in combining datasets from different groups. Camden relied on siloed information from a singular dataset, but the reality is that outside factors play a huge role in the overall health of a region’s population. Access to quality education and healthcare, economic stability, and other aspects of social equity can determine whether someone is more or less likely to suffer from a serious health condition.
These factors are commonly known as “social determinants of health,” but I think a more accurate term is “social informants of health.” Where someone lives doesn’t necessarily determine whether or not they’ll get sick or use drugs–but it can inform the likelihood they will be subject to these adversities. By looking at a variety of data sets–such as socioeconomic, health and community data–we can make better and more informed decisions that will positively impact people’s health.
Analytics are an imperfect but powerful tool
Data can be used to improve outcomes and create a healthier society, but we need to remember that there are no absolutes. Analytics can show us probabilities that something will happen. With more complete data, we can be reasonably certain that those probabilities will come to pass and take action to prevent them. Analytics, when used correctly, are a potent tool in the ongoing effort to help those in need.
Case Study
In this case study you’ll learn how Voyatek helped the Commonwealth of Virginia
- Develop, configure, and implement an easy-to-use data sharing system.
- Facilitate data-sharing across state agencies and local entities
- Perform advanced data analysis. including predictive analytics that involve the creation of risk profiles.
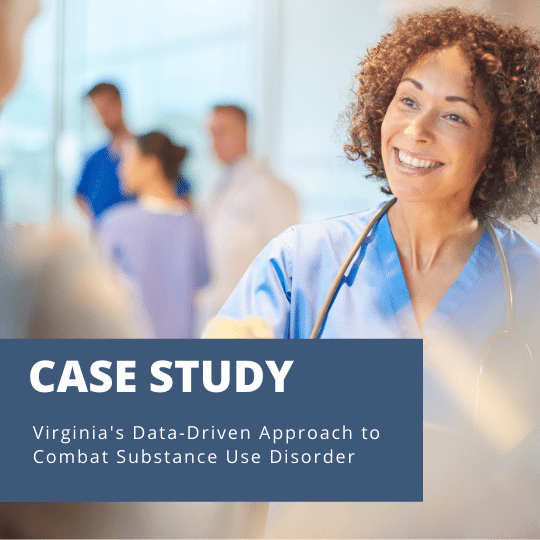